Let’s start with an insightful question for all marketers: Why do we advertise? The obvious answer is to promote our brand but are we really doing that by making ineffective ads? Delivering an ad is more complex than trying to assemble IKEA furniture with no instructions, a missing screw, and a cat determined to "help." Yes, it is not a child’s play and no, generic ads are not the way to go. One has to study the market, predict what their target audience is looking for, and get into the minds of the viewers.
This might have seemed overwhelming in the past but today, with AI, it is much easier and faster to achieve behavioral insights. For example, some AIs can predict how much attention your ad will get before you make it live! Such findings are a game changer and this is what predictive advertising is about: using data analysis and artificial intelligence to predict how people will respond to ads, based on their past behavior and preferences.
What is Predictive Advertising?
You now already know the gist of it, but let's dig deeper. Predictive advertising falls under the field of predictive analytics.
Predictive analytics is a broad technique that uses data and AI to forecast future events and behaviors across many industries, helping organizations make better decisions. Predictive advertising specifically applies these techniques to the realm of advertising, predicting how consumers will engage with ads to deliver more personalized and effective marketing messages.
How does it work?
Predictive advertising’s approach is exceptional and the results gained by it are insightful. This is an important section to understand how this even works. For simplicity, let’s categorize four different areas and see the workings of it.
Data Collection and Analysis
- Behavioral Data:
- Collects information about online activities, such as browsing history, purchase behavior, and social media interactions. This helps in understanding how users interact with content and predict future behaviors.
- Example: Analyzing a user’s frequent visits to a product page to predict a likely purchase.
- Demographic Data:
- Gathers details like age, gender, location, and income level to create a detailed customer profile. This helps in tailoring marketing messages to specific audience segments.
- Example: Targeting luxury goods to high-income demographics in specific locations.
- Contextual Data:
- Analyzes the context in which users engage with content, including the device used, time of day, and environmental conditions. This provides deeper insights into user preferences and situational behavior.
- Example: Displaying ads for coffee shops to users browsing in the morning on mobile devices.
Machine Learning and AI
- Pattern Recognition:
- Machine learning models sift through vast datasets to identify trends and patterns in user behavior. These insights help in understanding what drives consumer actions and preferences.
- Example: Identifying a trend where users who watch cooking videos are also likely to buy kitchen gadgets.
- Predictive Modeling:
- Uses historical data to forecast future actions. Predictive models estimate the likelihood of a user taking a specific action, such as making a purchase or clicking on an ad.
- Example: Predicting a user's likelihood to subscribe to a streaming service based on their past viewing habits.
Ad Targeting and Personalization
- Targeting:
- Ads are delivered to users who are most likely to be interested, based on predictive insights. This ensures that marketing efforts are focused on the most promising prospects.
- Example: Showing fitness equipment ads to users who frequently search for workout routines.
- Personalization:
- Ads are customized to match the preferences and behaviors of individual users, making them more relevant and engaging.
- Example: Displaying ads for sports shoes to a user who recently purchased athletic apparel.
Real-Time Optimization
- Dynamic Adjustment:
- Predictive advertising allows for continuous, real-time optimization of ad campaigns. If user behavior changes, the ad strategy adapts instantly to maintain effectiveness.
- Example: Adjusting ad bids automatically when a surge in interest for a product is detected.
- A/B Testing:
- Different versions of ads are tested to determine which performs best with various audience segments. This helps in refining and enhancing ad effectiveness.
- Example: Testing two ad creatives to see which generates more clicks among a target audience.
This shows how precise the advertisement has become which makes marketing more meaningful and less generic. Comparing predictive with traditional advertising is a better bridge to understanding what has changed and what yields the best results. The next section will unveil the differences between them.
What is the difference between predictive and traditional digital advertising?
The concept of "predictive advertising" might seem a bit redundant because, in reality, advertising has always been about predicting consumer behavior. Traditionally, marketers have relied on analyzing past data to forecast which segments of the audience are most likely to engage with their ads and offers. This approach involved using human judgment to interpret historical performance and make educated guesses about future responses.
However, the game has changed dramatically with the advent of AI-powered predictive advertising. Instead of relying on human intuition and manual analysis, machine learning algorithms now take the reins. These sophisticated models analyze vast amounts of data far beyond human capacity, uncovering patterns and trends that predict consumer actions with remarkable accuracy.
Before singing praises for predictive advertising, let’s first analyze what traditional digital advertising does and how it is different from predictive advertising
Traditional Digital Advertising
- Demographic Targeting:
- Traditional digital advertising primarily relies on broad demographic information, such as age, gender, and location. Ads are shown to large groups of people based on these basic characteristics, which often leads to a less personalized experience.
- Example: A clothing retailer runs a Facebook ad targeting women aged 25-40 in urban areas, promoting their new summer collection. The ad is the same for all women in this age group, regardless of their individual style preferences.
- Standardized Ads:
- Ads in traditional digital marketing are typically uniform across large audience segments. This approach means that the same ad is shown to many people, regardless of their individual preferences, resulting in a one-size-fits-all strategy.
- Example: A tech company launches a Google Ads campaign promoting their latest smartphone. The same ad appears for all users who search for "new smartphones," regardless of their specific interests or previous buying behavior.
- Static Campaigns:
- Campaigns are generally set up and run with limited adjustments. Once a campaign is launched, it follows a predetermined path with minimal changes, lacking the flexibility to adapt to new insights or changing audience behaviors.
- Example: A holiday resort launches a three-month advertising campaign promoting summer vacations. The campaign runs without any adjustments, even if data shows increased interest in the winter holidays.
- Reactive Approach:
- Traditional advertising responds to known consumer behaviors and preferences. It targets users based on what they have already shown interest in, rather than anticipating what they might want in the future.
- Example: A fitness brand targets users who have recently searched for "gym memberships" with ads for their latest subscription offers. They react to past searches but don't predict or influence future searches or needs.
Key Differences: Predictive Advertising
- Targeting Precision:
- Predictive Advertising: Uses advanced data analytics, machine learning, and AI to make sophisticated predictions about each user's future behavior and preferences. This allows for more precise targeting of ads to individuals likely to be interested in them.
- Traditional Advertising: Targets ads based on broad demographic categories and past behaviors, which often leads to a less tailored approach.
- Example: An online bookstore uses predictive advertising to recommend specific books to users based on their past reading habits and browsing history. If a user frequently buys science fiction novels, the ads will feature the latest sci-fi releases tailored to their taste.
- Personalization:
- Predictive Advertising: Delivers highly personalized ads that align with individual users’ predicted interests, creating a more engaging and relevant experience.
- Traditional Advertising: Offers more generalized ads that may not resonate as well with specific users due to their broad targeting criteria.
- Example: A fitness app uses predictive advertising to show personalized workout gear ads based on each user's exercise preferences and past purchases. Users who often search for yoga-related content will see ads for yoga mats and apparel.
- Campaign Adaptability:
- Predictive Advertising: Allows for real-time adjustments and optimizations based on ongoing data analysis, ensuring campaigns remain relevant and effective.
- Traditional Advertising: Typically involves fewer adjustments, with campaigns often running as initially planned without significant changes.
- Example: Netflix uses predictive advertising to promote new shows. If real-time data shows that a particular show is trending among younger audiences, the platform dynamically adjusts the campaign to target more ads toward that demographic.
- Data Utilization:
- Predictive Advertising: Leverages large datasets and complex algorithms to foresee and influence future consumer behavior. It uses a wide range of data points to anticipate what users will want next.
- Traditional Advertising: Utilizes simpler data and metrics, focusing on reaching a broad audience based on past behaviors and static criteria.
- Example: A luxury hotel chain uses predictive analytics to identify potential high-value guests. They analyze past booking data, browsing history, and social media engagement to target ads promoting premium suites and exclusive experiences to users likely to be interested in luxury travel.
- Strategic Approach:
- Predictive Advertising: Adopts a proactive strategy, engaging users before they even search for something. It anticipates needs and preferences, positioning brands ahead of consumer actions.
- Traditional Advertising: Follows a reactive strategy, targeting users based on known interests or behaviors, without the foresight into what they might need or want next.
- Example: A grocery delivery service anticipates a rise in demand for comfort foods during a cold snap. They proactively adjust their advertising to highlight hot soups and hearty meals before users start searching for these items.
To put it simply, predictive advertising is like having a personal shopper who intuitively knows your tastes and suggests products you’ll love before you even ask. In contrast, traditional digital advertising is more like a billboard that everyone driving by can see, regardless of whether they are interested in the advertised product or not.
By leveraging predictive advertising, businesses can create more targeted, personalized, and adaptable campaigns that resonate with their audience's future needs and preferences, offering a significant advantage over traditional methods.
How can you use it to transform your marketing?
Using predictive advertising is as easy as setting up your campaign preferences and letting the algorithms do the rest. All you have to do is research the right tools to get started. Choosing the right tool depends on your needs:
- Starting Out: If you're new to predictive advertising, Google Ads can be a good starting point due to its user-friendly interface and wide reach.
- Advanced Targeting: For highly targeted and personalized ad campaigns with a larger budget, consider Demand-Side Platforms (DSPs).
- Industry Specificity: Certain industries might have specialized platforms for predictive advertising within their niche.
To give you a brief overview of the options mentioned above are:
Google Ads is a popular online advertising platform that lets businesses create and manage ads across various Google products like Search and YouTube. While not the most advanced tool for predictive advertising, can still be useful in a few ways:
- Limited Predictive Targeting: It offers some features for targeting based on search queries related to future needs (e.g., someone searching for "pregnancy tips" might see baby product ads).
- Large User Base: Reaches a massive audience across Google Search and other properties, giving you a wider pool of potential customers.
- User Search Intent: Allows targeting based on keywords people are actively searching for, which can provide clues about their present and potentially near-future needs.
However, it's important to remember that Google Ads focuses more on past behavior and demographics compared to advanced predictive advertising platforms.
Demand-side platforms (DSPs) act like a high-tech matchmakers for advertisers and potential customers. Instead of relying on broad demographics, DSPs consider a vast amount of user data, including browsing habits, app usage, and even offline purchase history (with permission). By analyzing this data with machine learning, DSPs predict what a user might be interested in before they even start searching for it. This allows advertisers to target these users with relevant ads at the exact moment they're most receptive, increasing the effectiveness of their campaigns.
Industry-specific platforms are becoming popular as they use innovative methods of predictive advertising. One such company is alpha.one which has introduced two predictive platforms called expoze.io and junbi.ai. Predictive Advertising with alpha.one:
1. AI-Powered Insights:
- junbi.ai and expoze.io utilize AI to predict ad performance, focusing on how ads will engage viewers and which elements will draw the most attention.
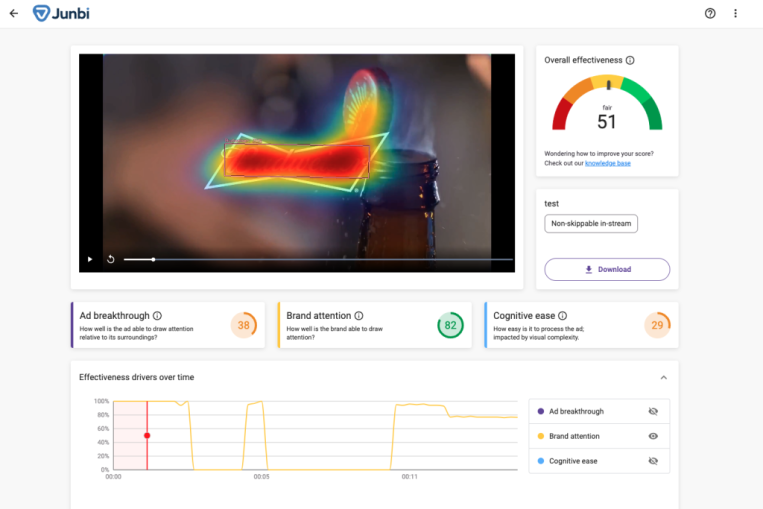
2. Data-Driven Optimization:
- These tools analyze data to provide actionable insights for improving ad content, ensuring higher effectiveness and engagement pre-launch.
3. Efficiency and ROI:
- By predicting outcomes, alpha.one helps reduce wasted ad spending and increases return on investment, making advertising campaigns more efficient and impactful.
Key Benefits:
- Informed Decisions: Based on predictive analytics.
- Rapid Adjustments: Quick feedback for continuous improvement.
- Creative Enhancement: Experimentation with different elements.
By focusing on these aspects, alpha.one exemplifies the transformative power of predictive advertising in modern marketing strategies.
Conclusion
Predictive advertising is more than just a buzzword—it's a game-changer for modern marketers. By harnessing the power of AI and data analytics, you can move beyond generic, one-size-fits-all campaigns to create personalized ads that truly resonate with your audience. Imagine being able to predict what your customers want before they even know it themselves, tailoring your messages to fit their exact needs and preferences. This not only boosts engagement and effectiveness but also maximizes your ROI by ensuring every ad dollar is well spent. As the digital landscape continues to evolve, integrating predictive advertising into your strategy is essential for staying ahead of the curve and forging deeper connections with your audience. Embrace this cutting-edge approach, and watch your ad campaigns transform from ordinary to extraordinary.